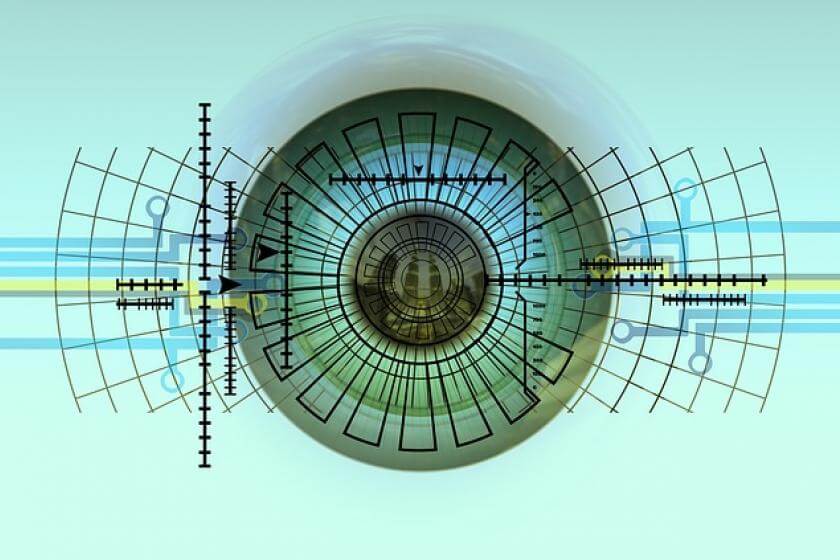
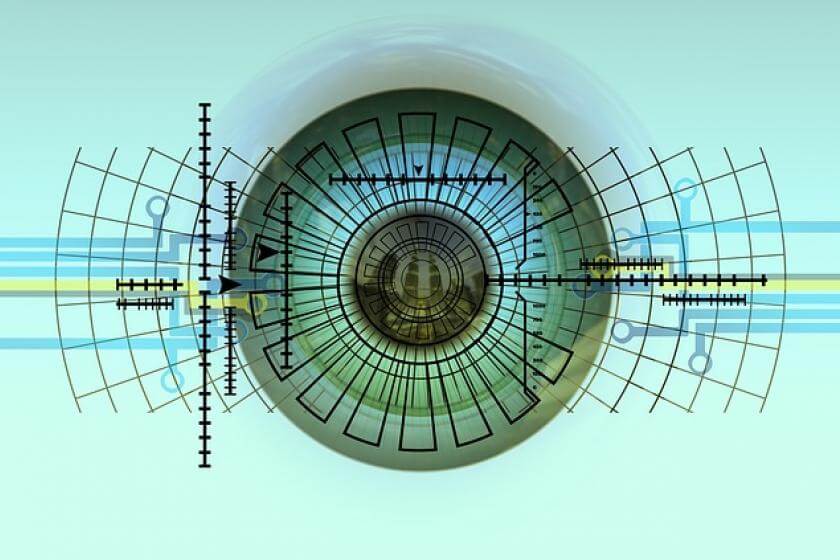
- Details
- Published on 23 August 2021
(Source: Pixabay)
As results, performance factors, and challenges come to light, interest spreads for IT managers.
The Olympics in Japan will use facial recognition this summer. And Disney World announced in May it will test the technology for a month to cut wait times at the theme park as part of a more touchless experience.
But before getting serious about cloud-based or on-premises facial recognition systems, IT managers must first determine whether they will meet the desired performance levels required by the enterprise in its application.
Often lost in the heated debate over whether municipalities and law enforcement entities should be allowed to use facial recognition to identify suspects in crimes is a secondary question; How effective are the AI-driven, software-based systems?
And before we can dig into early returns on performance, we must acknowledge that there are far more verticals that could apply facial recognition – beyond enterprises with access control implementations, law enforcement organizations, and large multipurpose sports venues looking beyond identifying staff.
Vertical value
- Retail. Identify shoplifters to reduce shrinkage.
- Transportation. Airport security. Reduce waiting times. Check-in kiosks.
- U.S. government. Deploy for border control uses.
- Entertainment. Security for mass events at large venues such as the Olympics, etc.
- Banking. Replace passwords with screen-based face scanning for online banking.
- Healthcare. Deploy touchless access to secure lab gear, clean rooms, and more.
- Media/marketing. Test audience reaction to movie trailers, characters in TV pilots, and optimal placement of TV promotions.
- Consumer products. Measure visual emotions through facial analysis to pick candidates for new or enhanced offerings.
- Travel. The US Department of Homeland Security predicts that facial recognition will be used on 97% of travelers by 2023.
Performance, historically
How far have facial recognition systems come? The Technology Policy Program at the Center for Strategic and International Studies (CSIS) produces non-partisan and non-proprietary research, took a look.
Facial recognition has improved dramatically in only a few years, according to CSIS blog author William Crumpler in April 2020. “As of April 2020, the best face identification algorithm has an error rate of just 0.08% compared to 4.1% for the leading algorithm in 2014, according to tests by the National Institute of Standards and Technology (NIST). As of 2018, NIST found that more than 30 algorithms had achieved accuracies surpassing the best performance achieved in 2014.”
But beyond algorithms, how are the systems faring in real-life deployments in the last few years? The answer, of course, is based on how its results are used, not necessarily measured.
Beyond the AI engine
A case in point is the New York Police Department (NYPD), which began a massive storage upgrade in 2009 to support the deployment of its facial recognition systems in parts of the Big Apple, such as the financial district. However, it is important to note that results that the entity released last year are used as one piece of a process for which its owner assesses the effectiveness.
The NYPD has long stated that any “matches” are used as only one part of an investigation. They are just leads and NOT enough to issue arrest warrants for. And before it becomes a useful lead for criminal investigators, a match from facial recognition is analyzed by a group that reviews each and weighs in on the quality – and hence viability - of the match.
Further review
“If possible, matches are identified, then trained Facial Identification Section investigators conduct a visual analysis to assess the reliability of a match and conduct a background check to compare available information about the possible match and relevant details of the investigation,” according to the NYPD FAQ.
Specifically, the NYPD uses facial recognition to compare images obtained during criminal investigations with lawfully possessed arrest photos. When used in combination with human analysis and additional investigation, facial recognition technology is a valuable tool in solving crimes and increasing public safety.
The NYPD measures the effectiveness of its facial recognition software on how it has done in terms of providing substantial leads in criminal cases. Last year, the NYPD released results for the 2019 calendar year. The organization explained that following; “(Its) Facial Identification Section received 9,850 requests for comparison and identified 2,510 possible matches, including possible matches in 68 murders, 66 rapes, 277 felony assaults, 386 robberies, and 525 grand larcenies. “The NYPD knows of no case in New York City in which a person was falsely arrested on the basis of a facial recognition match,” it added.
What affects facial recognition performance
Those in the know cite three variables that can impact the accuracy of a facial recognition system’s ability to make matches. They are the person’s pose, illumination, and expression (PIE) in the photo.
The mask factor
And perhaps not much of a concern before the global pandemic was the use of face masks. It is unclear what the long-term prospects for facemasks use after COVID-19 in the U.S and elsewhere are. But they have left their mark.
Consider this research and testing from NIST. The entity tested 89 commercial facial recognition algorithms. It used digitally applied masks and claimed to have found a 5-50% error rate in matching faces with them to photos of the same person.Vendors are hard at work improving that error rate. BBC reported that a US Department of Homeland Security "controlled-scenario test" in January found one facial recognition system with a 96% success rate - although the results of those tested "varied greatly."
Environment dependent
And while it is a safe bet that we will see less mask-wearing, save for the winter months, some facial recognition systems will stay have to deal with what is known as facial occlusions. They include longtime items as hats, sunglasses, scarves, and heavy facial hair. These items are known to weaken the performance of facial recognition systems, especially when they are used in applications that use video surveillance.
IT managers with controlled access scenarios in mind for their systems may not need to be concerned with this variable in facial recognition accuracy.The road ahead
Facial recognition systems are attracting broader interest in the form of tests and actual deployments, by users including Disney World and the 2021 Olympics. But deployments that include the municipal law enforcement entities face headwinds from those concerned with privacy and Big Brother concerns.